Feature Selection Techniques for CR Isotope Identification with the AMS-02 Experiment in Space
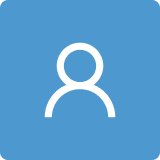
Round 1
Reviewer 1 Report
Comments and Suggestions for AuthorsThe paper is interesting and well written. Because it is more concerned about methods rather than about a scientific finding, its main purpose is to explain a technique so it can be adapted and replicated to other cases. With this mindset, I propose the following additions:
1) Add an appendix with the listing of all 130 features, each with few explanatory words. If a reader wants to figure out how to tackle his/her case, a detailed comparison with the proposed case is much more meaningful than just reading that a generic set of variables was more effective and others performed less well.
2) Make quantitative statements about computational complexity/CPU wall time. Trade-offs of quality vs. speed with make sense if they are quantitatively justified, at least by orders of magnitude.
3) I agree with the purpose of avoiding overfitting, but did you actually detect overfitting if using the full set of variables? Does the final set really avoid overfitting? Can this be shown quantitatively?
In page 9, Figure 4 is almost impossible to read for people with partial or full colour-blindness. It is beautiful, but I have concerns about this way of presenting data, as the lines connecting the data points carry no meaning at all. A representation like in Figure 5 would be less fancy but much easier to understand.
Finally, I think I spotted a couple of typos:
Page 12, line 379, Figures->Figure
Page 12, line 380, lines -> rows
All in all, it's a nice job, and I think all the work done can be better appreciated if the communication is improved as suggested.
Author Response
Please see attachment.
Author Response File: Author Response.pdf
Reviewer 2 Report
Comments and Suggestions for Authors- Check punctuation marks and capital letters after equations.
- In my opinion several parameters are reported with too many significant figures.
Author Response
Please see the attachment.
Author Response File: Author Response.pdf
Reviewer 3 Report
Comments and Suggestions for AuthorsThe precise spectral measurements of AMS-02 experiment shed new light on the cosmic ray propagation. In this work, the authors propose feature selection techniques base on machine learning to perform CR isotope identification with the AMS-02 experiment. The topic is very interesting and has very high physical significance. But the author should address my questions and provide significant improvements before I make a decision for publication or not.
1. The major problem is that the author should clearly describe their own contribution, which is different with other works. In my understanding, this work only perform five algorithms comparison and gain the better result. Based on this alone, the lack of originality in this work is not sufficient for publication. The authors should Please provide a concise summary of the main contributions of this work.
2. Fig.5 and table 2 give the comparison results. Clearly, Kbest, RF, Correlation and All is similar in accuracy, precision, F1-core, recall. But is larger difference in p-value. Please carefully examine the results and provide a detailed description of them in the article.
3. The AMS-02 data December 9, 2015, to May 9, 2016, were used in this work. Why only use this special data set.
4. To more convincing, can the author present the real data results about those methods? In one word, did the techniques mentioned in the article really have an impact on the original data?
Author Response
Please see the attachment
Author Response File: Author Response.pdf
Reviewer 4 Report
Comments and Suggestions for AuthorsThe proposed work focuses on feature selection techniques for ML models dedicated to isotope identification. In particular, the paper is aimed at enhancing the classification performance of Boosted DTs applied for RICH background reduction.
Given that the performance of Boosted DT models critically depends on the input features used during the training phase, the authors compare in this work five different feature selection algorithms.
General comment:
The paper is well-written and easy to follow. The goal of the paper is clear and the manuscript has been written accordingly.
My main concerns are about the methodology and the reason behind the work. Basically, the feature selection phase is one of the preliminary steps of any ML workflow, together with the data cleaning and normalisation and the model selection and tuning. And the estimation of the most relevant features to be kept for the predictors’ training may be performed according to several strategies, as mentioned by the authors. These strategies are already implemented in several free libraries, as Python’s scikit-learn. As a result, the feature selection process may be fast and require little human effort. Also the comparison of different alternatives may be performed automatically. For this reason, the paper appears as a trivial set of experiments for a given case study, without any actual advancement for the ML theory or in the feature engineering area. Furthermore, the paper presents no particular ML applications that may be exploited by the community, nor breakthrough results obtained via ML predictors. According to these considerations, the paper appears as lacking of novelty and significance, and thus it is deemed not suitable for the publication in this journal.
Other comments:
Section 2 is (in my opinion) too much detailed, since the provided details are not very relevant to understand or to justify the presented work.
Conversely, Section 3 contains some trivial information, e.g., the explanation and formal definition of mainstream ML classification metrics. The section is also redundant, since it is common to adopt only a single classification score to evaluate ML models, e.g., the F1 score (given that it considers both precision and recall, and it is usually correlated with the accuracy score). [Fig. 5 depicts this observation].
The experimental section should be improved. When using the k-best method, the authors should specify the adopted value of k and present how the performance varies by changing the k value, since the performance is strictly dependent on k. Analogously, why the linear regression approach considers only one feature? Which kind of threshold has been used on the identified coefficients? Which are the results by varying this threshold? [These missing experiments may impact on the opening statement of Section 4.4].
Another missing experiment in Section 4 is to adopt a destructive approach on the feature set, e.g., training a Boosted DT with all the input features, checking its predictive performance and then removing one feature at a time, iteratively, to find the least impacting on the overall classification performance. This may be a time-consuming task, given the amount of features, but completely automated and customisable by imposing a threshold on the maximum allowed performance decrease w.r.t. the initial classification performance.
Finally, I believe that the work could be presented in a more general way, since the selection of a proper set of input features is beneficial not only for BDTs, but also for other ML models.
Minor:
In Fig. 2 I believe that the absolute values for the clusters would be more interesting and readable than the percentages, as already done in the text.
Please, check the sentence at line 221 and the punctuation at line 424.
There are a missing ; before email address in affiliation 3 and a missing blank space between the third author and the corresponding affiliation number.
There is a mismatch between the paper title and the citation suggested in the first page (left sidebar).
Please, check the Author Contributions statement.
Please, also check in-text citations (some lack a blank space before the brackets).
Percentages in Table 1 are not always correct.
p-values should be included in Fig. 5, since all the others indices reported in Table 2 are shown.
Author Response
Please see the attachment
Author Response File: Author Response.pdf
Round 2
Reviewer 3 Report
Comments and Suggestions for AuthorsMany thanks to the authors for answering all my questions thoroughly. For my, it can be published in current version.